Instance-guided Cartoon Editing with a Large-scale Dataset
Caritas Institute of Higher Education
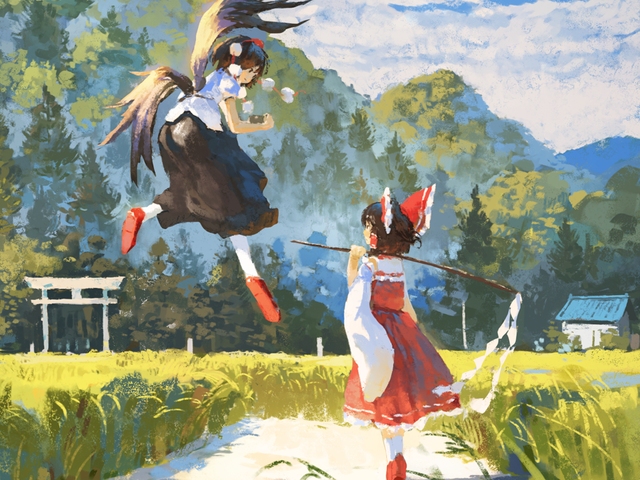
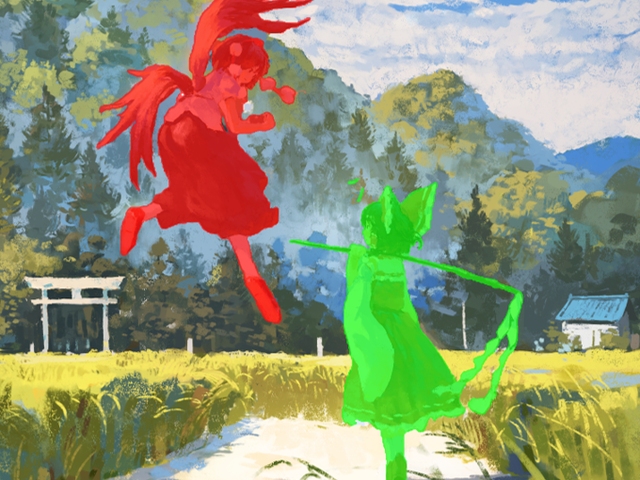
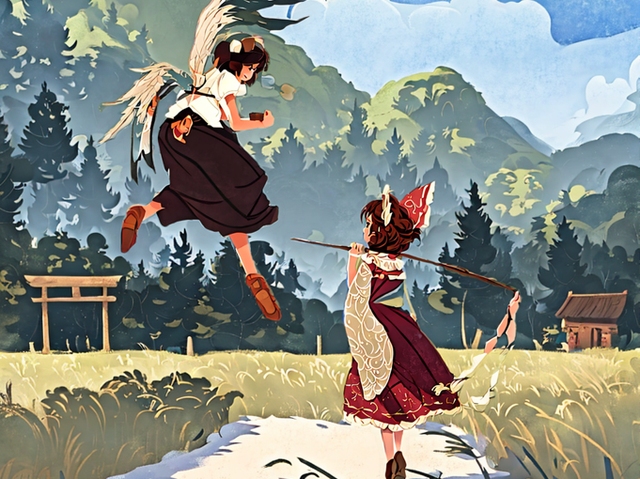
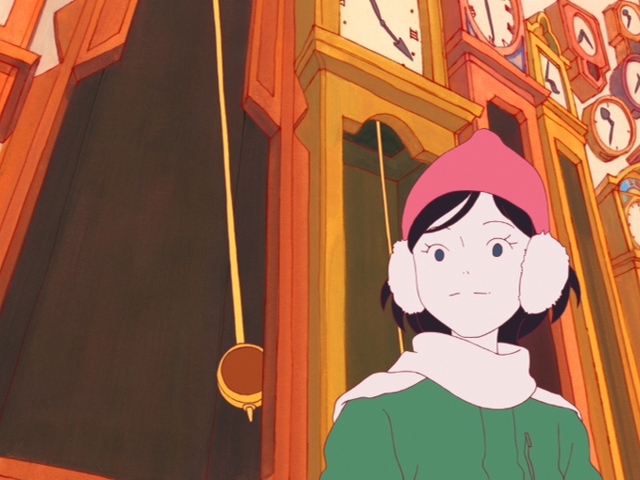
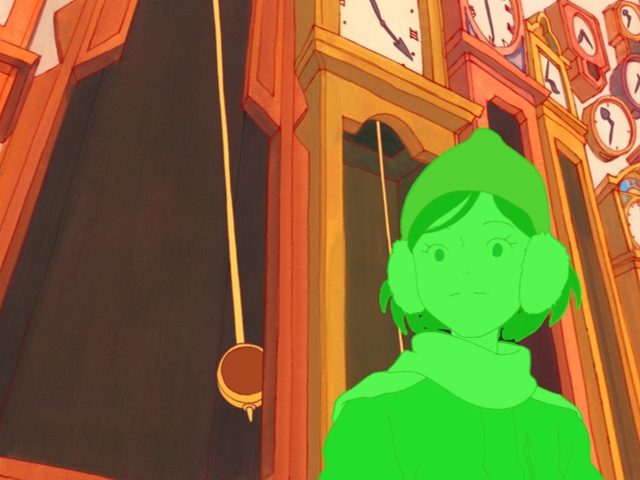
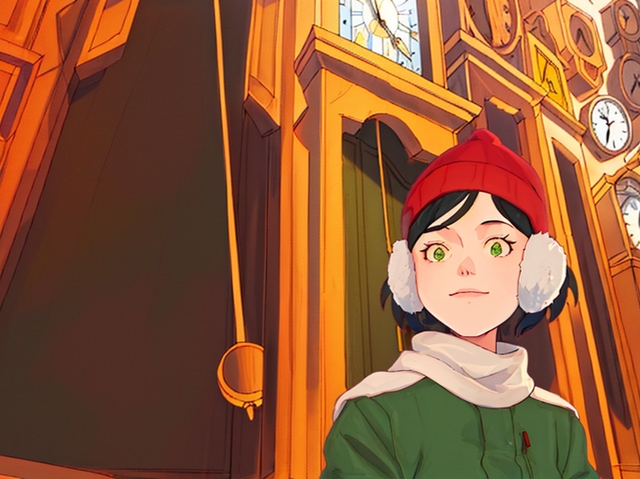
- [Arxiv]
- [Code]
- [HuggingFace] (coming soon)
- [Colab] (coming soon)
Caritas Institute of Higher Education
Abstract
Cartoon editing, appreciated by both professional illustrators and hobbyists, allows extensive creative freedom and the development of original narratives within the cartoon domain. However, the existing literature on cartoon editing is complex and leans heavily on manual operations, owing to the challenge of automatic identification of individual character instances. Therefore, an automated segmentation of these elements becomes imperative to facilitate a variety of cartoon editing applications such as visual style editing, motion decomposition and transfer, and the computation of stereoscopic depths for an enriched visual experience. Unfortunately, most current segmentation methods are designed for natural photographs, failing to recognize from the intricate aesthetics of cartoon subjects, thus lowering segmentation quality. The major challenge stems from two key shortcomings: the rarity of high-quality cartoon dedicated datasets and the absence of competent models for high-resolution instance extraction on cartoons. To address this, we introduce a high-quality dataset of over 100k paired high-resolution cartoon images and their instance labeling masks. We also present an instance-aware image segmentation model that can generate accurate, high-resolution segmentation masks for characters in cartoon images. We present that the proposed approach enables a range of segmentation-dependent cartoon editing applications like 3D Ken Burns parallax effects, text-guided cartoon style editing, and puppet animation from illustrations and manga.
3D Ken Burns
Style Editing
Project Video Walkthrough
BibTeX
@article{animeins, Author = {Jian Lin and Chengze Li and Xueting Liu and Zhongping Ge}, Title = {Instance-guided Cartoon Editing with a Large-scale Dataset}, Eprint = {2312.01943v1}, ArchivePrefix = {arXiv}, PrimaryClass = {cs.CV}, Year = {2023}, Month = {Dec}, Url = {http://arxiv.org/abs/2312.01943v1}, File = {2312.01943v1.pdf} }
Project page template is borrowed from DreamBooth.